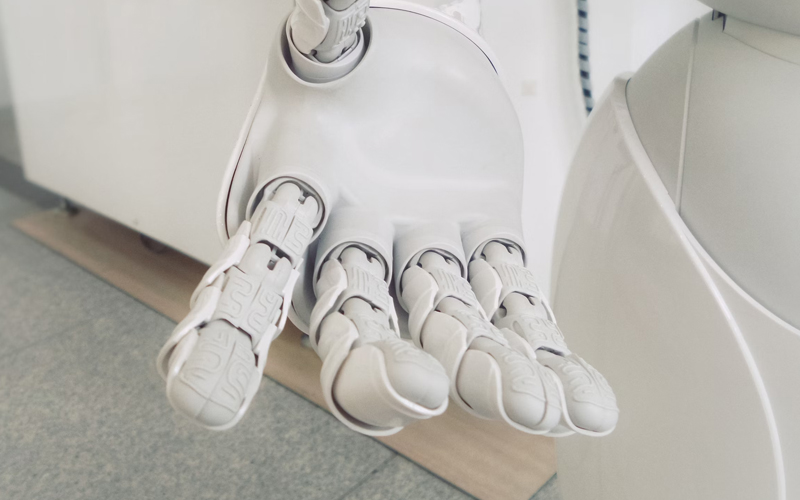
The experiment with a four-legged robot balancing on a fitness ball demonstrates much more than it may seem at first glance. In fact, it showcases the power of artificial intelligence, such as GPT-4, to train robots to perform complex tasks in the real world much more efficiently than humans. The NewAtlas portal drew attention to this topic.
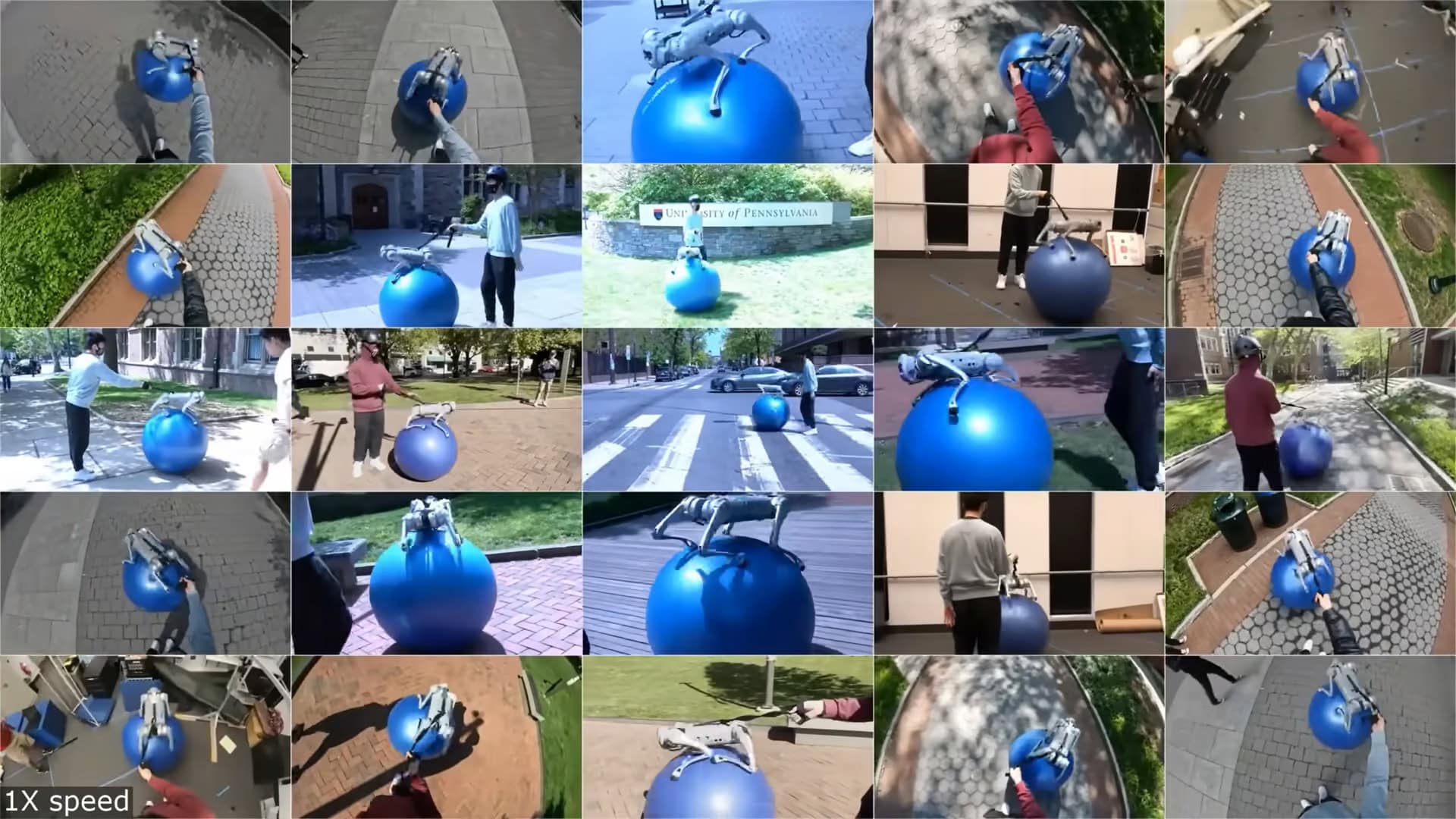
An innovative software called DrEureka, powered by the capabilities of large language models like GPT-4, brings a revolution in the field of training robots to perform complex tasks in the real world. The foundation of the DrEureka project is the so-called “sim-to-reality” method, which acts as a bridge between virtual simulation and physical demonstration.
A perfect example is the four-legged robotic “dog” balancing on a fitness ball and gradually moving forward on its surface. While it may seem like just an engineer’s gimmick at first glance, it actually demonstrates impressive training possibilities driven by artificial intelligence.
Jim Fan and his team developed an open-source software called DrEureka, where “Dr” refers to “domain randomization,” where variables such as friction, weight, center of mass, and similar parameters are randomly changed in a simulated environment.
For this experiment, experts utilized the four-legged robot Unitree Go1, priced at around $6000. During the simulation in the DrEureka environment, the robot was rewarded for each successful attempt and punished for each unsuccessful attempt. This reward process allowed the robot to experiment with various techniques and strategies without the risk of damage.
After training in the virtual world, a test followed in the real world, where the device had to demonstrate its abilities acquired during the simulation.
According to scientists, DrEureka is the first software of its kind, capable of seamlessly transitioning from simulation to the real world thanks to the “sim-to-reality” method without prior attempts. According to researchers, the entire system could be significantly improved if feedback from the real world could be provided through GPT, not just reading error records.
In other words, if GPT could actually see what happened in the real world (e.g., through video) and not just read error logs, the entire training process could be even more effective.